Math professor awarded NSF grant for research
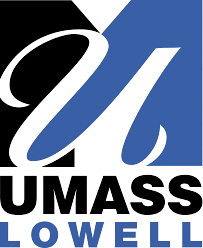
LOWELL, Mass. – A UMass Lowell professor recently received a grant from the National Science Foundation (NSF) to explore how change points affect statistics.
Nilabja Guha, assistant professor of mathematical sciences, received a three-year, $140,000 NSF grant for his project, “New Directions in Bayesian Change-Point Analysis,” which aims to fill a gap in the statistical tools used in the analysis of changes in data.
In his research, Guha will use the Bayesian method – a mathematical technique that applies probabilities or distributions to statistical problems and allows people to revise and update their assumptions after obtaining new experimental data – to develop methodologies for analyzing changes in the underlying data-generating process.
According to Guha, almost all dynamic and random processes in nature go through sudden and significant structural changes. Often, the change is expressed in an observable quantity, such as the change in fuel prices, stock indices, crime activities or population density, which responds significantly to a change in an unobservable factor such as an economic downturn, a change in public policy or an outbreak of disease.
“Such change points are routinely observed across all scientific disciplines and applications, including economics, epidemiology, social sciences, cybersecurity, finance and others,” he said.
Guha noted that while there is substantial literature proposing elaborate methods for detecting change points in different settings, there has been limited consideration of change points in what he considers as “hierarchical models with complex dependence or sparsity structures.”
“My research fills this gap with new statistical tools motivated by specific, real-life applications by developing a theoretical framework while retaining efficiency and usefulness in current applications,” he said.
Through the project, Guha hopes to outline a comprehensive framework for estimating the change point in problems that may arise in different applications.
“They can include change points in data with massive dimensions, such as those one would encounter in, say, high-resolution imaging or complex connected graphs,” he said.